The Importance of Data Labeling Tools in Today's Business Landscape
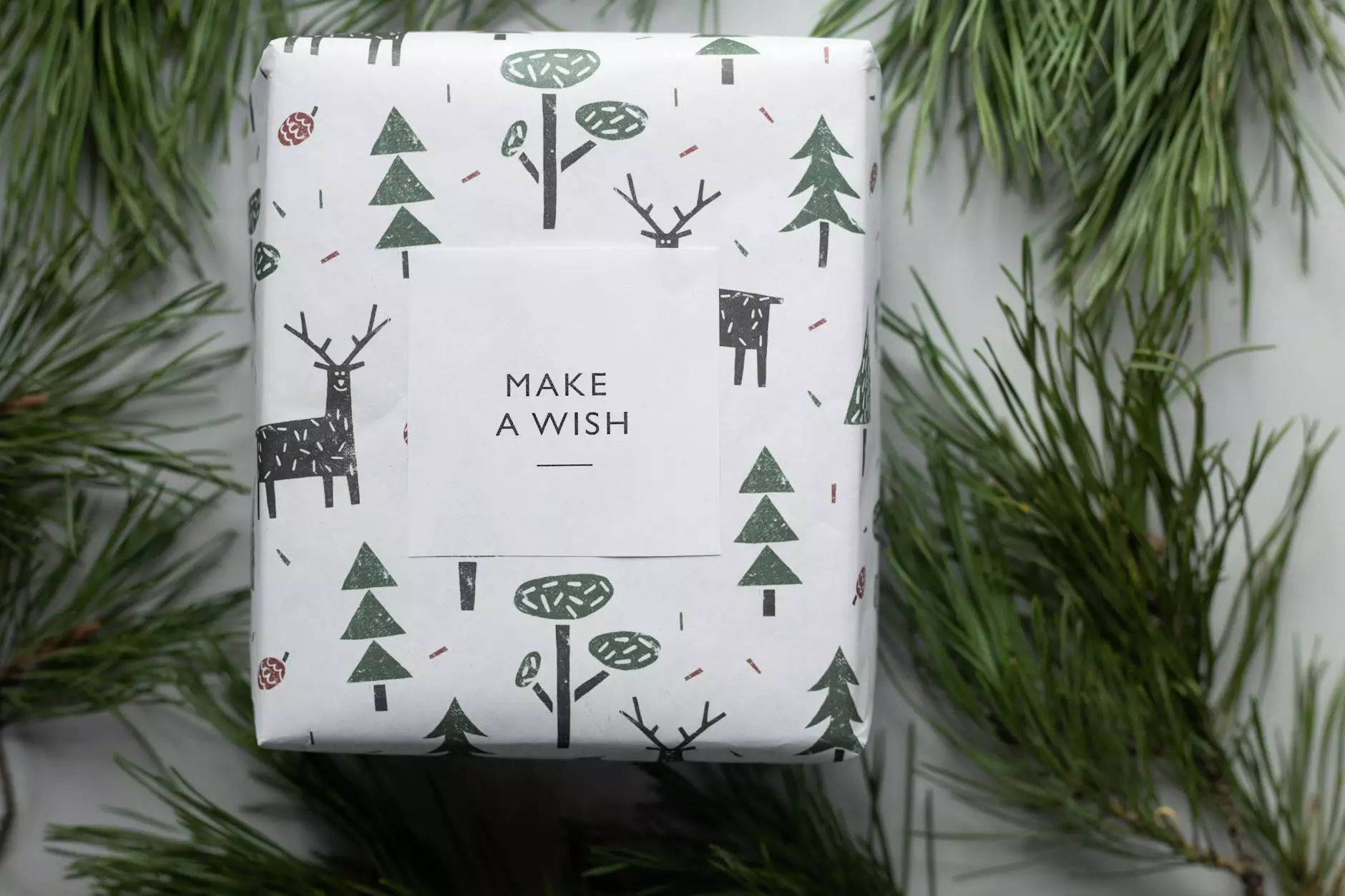
In an era where data drives decision-making and innovation, businesses increasingly rely on accurate data to fuel their artificial intelligence (AI) and machine learning (ML) initiatives. Central to these processes are data labeling tools, which transform raw data into meaningful information. This article explores the role of data labeling tools in the business landscape, focusing on how they enhance productivity and improve outcomes.
What Are Data Labeling Tools?
Data labeling tools are software applications designed to annotate, categorize, and structure data. This process is crucial for training machine learning models, as it allows algorithms to recognize patterns and make informed predictions based on data inputs. In practical terms, data labeling involves tagging images, text, audio, or video content so that AI systems can learn from them effectively.
Types of Data Annotation
Data labeling can vary significantly based on the type of data being processed. Here are some common forms of data annotation:
- Image Annotation: Involves tagging objects within images, such as identifying vehicles in traffic signs for autonomous driving systems.
- Text Annotation: Deals with identifying sentiment, entities, or intent within textual information, which is essential for natural language processing (NLP) applications.
- Audio Annotation: Involves transcribing and labeling audio clips, often used in voice recognition systems.
- Video Annotation: Entails tagging moving objects in video content for applications like surveillance and smart city infrastructure.
The Business Need for Data Labeling Tools
As organizations increasingly adopt AI solutions, the demand for well-labeled datasets has skyrocketed. The following are key business needs for implementing data labeling tools:
1. Enhanced Accuracy
Data labeling tools significantly improve the accuracy of machine learning models. When datasets are labeled correctly, AI systems can learn with higher fidelity, thus reducing errors in predictive analytics. For instance, a well-annotated dataset can make the difference between a successful predictive maintenance program and a costly failure.
2. Faster Development Cycles
In the competitive landscape of technology development, being fast and efficient is paramount. By leveraging data labeling tools, businesses can expedite their data preparation process, enabling quicker deployment of AI solutions. With automated or semi-automated labeling systems, companies can significantly cut down the time it takes to prepare datasets, leading to faster go-to-market strategies.
3. Scalability of Operations
As businesses grow, their data needs evolve. Robust data labeling tools offer scalability, allowing organizations to handle larger volumes of data without compromising quality or accuracy. This scalability is crucial in industries where data is generated at an exponential rate, such as social media, financial services, and healthcare.
Key Features of Effective Data Labeling Tools
Not all data labeling tools are created equal. When selecting a suitable tool, businesses should consider the following features:
1. User-Friendly Interface
An intuitive interface is essential for maximizing productivity. A user-friendly platform ensures that users can easily navigate the tool, making the labeling process more efficient. Furthermore, the onboarding process for new team members becomes less cumbersome, driving quicker integration into ongoing projects.
2. Customization Options
Every business has unique data requirements. Effective data labeling tools allow for customization to suit specific labeling tasks. From creating unique tags to defining specific annotation guidelines, customization ensures that the tool meets the unique needs of the project.
3. Collaboration Features
Data labeling often requires input from multiple stakeholders. Tools that enable collaboration facilitate smoother workflows, allowing teams to work in tandem. Features such as real-time commenting, version control, and shared access can enhance teamwork.
4. Quality Control Mechanisms
To maintain high standards, effective data labeling solutions should incorporate quality control measures. These can include features like double-checking annotations, automated consistency checks, and the ability to track user performance over time. Ensuring data quality is vital for the success of any AI initiative.
5. Integration Capabilities
Integration with existing systems and data pipelines is essential for a seamless workflow. Tools that support integration with databases, machine learning frameworks, and cloud services enable businesses to leverage their existing infrastructure and minimize disruptions.
How Keylabs.ai Revolutionizes Data Annotation
At the forefront of the data annotation landscape is Keylabs.ai. This innovative platform provides a comprehensive suite of data labeling tools designed to meet the diverse needs of businesses. Here’s how Keylabs.ai stands out:
1. Advanced AI-Powered Labeling
Keylabs.ai utilizes advanced machine learning algorithms to aid in the annotation process. The platform’s AI capabilities help semi-automate labeling tasks, speeding up the process while maintaining accuracy. This feature is particularly beneficial for large datasets, where manual labeling can be time-consuming.
2. Extensive Support for Various Data Types
Whether you’re working with images, text, audio, or video, Keylabs.ai has got you covered. With tailor-made tools for each data type, businesses can ensure that their datasets are labeled appropriately for the specific requirements of their projects.
3. Scalability and Flexibility
The platform’s architecture is designed for scalability, enabling businesses to scale their labeling processes as data volumes increase. Flexibility in working with various projects and data sources ensures that Keylabs.ai adapts to your business needs.
4. Robust Analytics and Reporting
Effective data labeling is only part of the equation. Keylabs.ai offers robust analytics and reporting features, providing insights into labeling progress, quality scores, and user contributions. This data is invaluable for ongoing process improvements and identifying areas for further training.
Real-World Applications of Data Labeling Tools
The impact of data labeling tools extends across various industries. Here are some real-world applications that highlight their significance:
1. Autonomous Vehicles
Self-driving technology heavily relies on accurately annotated data to navigate safely. Image data must be labeled to identify road signs, pedestrians, and obstacles. Efficient data labeling tools ensure that these datasets are prepared accurately and at scale, crucial for the advancement of autonomous vehicles.
2. Healthcare Technologies
In healthcare, data labeling tools help in medical image analysis, patient record classification, and even drug discovery processes. Accurate data labels enable algorithms to identify diseases and recommend treatment plans effectively, significantly improving patient care.
3. E-commerce Product Recommendations
E-commerce platforms utilize data labeling tools to enhance customer experiences through personalized recommendations. By labeling product descriptions, customer reviews, and behavior patterns, businesses can improve their recommendation engines to drive sales and customer satisfaction.
The Future of Data Labeling Tools
The future of data labeling tools is bright, driven by advancements in AI and machine learning technologies. As AI capabilities continue to evolve, so too will the sophistication of data labeling tools. Key trends to watch include:
1. Increased Automation
Automation will play a critical role in the future of data labeling. As algorithms improve, more aspects of the labeling process will become automated, reducing the reliance on manual input and driving faster turnaround times.
2. Integration of Augmented Intelligence
Tools that integrate augmented intelligence will empower human labelers to work more effectively alongside AI systems. This collaboration is expected to yield better quality annotations while allowing teams to focus on more complex tasks.
3. Growth of Synthetic Data
The rise of synthetic data will further influence data labeling processes. As organizations utilize generated data for training models, data labeling tools will need to adapt to accommodate this new type of input, ensuring that synthetic datasets are appropriately annotated.
Conclusion
As data becomes the cornerstone of decision-making and innovation, the significance of data labeling tools cannot be understated. Businesses that invest in quality data annotation processes render themselves better equipped to harness the power of AI and machine learning. With platforms like Keylabs.ai leading the way, organizations can ensure their data is prepared accurately and efficiently, setting the stage for successful AI initiatives and a competitive edge in today's data-driven landscape.